Many factors affect a company’s ability to service its customers and optimize the supply chain. One of the key building blocks in achieving those goals is having a reasonably accurate prediction of demand, including: (a) what goods will be demanded, (b) how much of each item will be demanded, (c) when the goods will be demanded, and (d) where the items need to be at the time they are demanded.
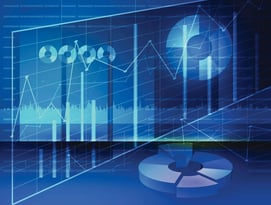
Demand Planning is the nexus of collaborative activity across multiple areas within the organization, with the goal of producing a reliable and accurate prediction of demand. It’s the process of gathering, interpreting, and evaluating intrinsic and extrinsic information, and formulating it into a prediction of future demand. Done well, demand planning transforms a reactive process (where the forecast adjusts based on the latest demand) into a proactive process (where the forecast anticipates events yet to occur).
A demand plan typically starts with a statistical forecast using historical data, and then has additional intelligence layered onto it: causal events, experimental methods, and judgmental factors. Typically the horizon is eight to eighteen months, with an emphasis on the lead time horizon. The resulting demand plan is an input to other business processes such as production scheduling, S&OP(Sales & Operations Planning), or SIOP(Sales, Inventory, and Operations Planning).
The foundation of the demand plan is statistical analysis of demand history. At the inception of Demand Planning in the organization, some important fundamental decisions must be made regarding the input data, such as what data belongs in the time series; the structure of the data; the means of data collection; and the timing and frequency of updating the data. These decisions are crucial, for the better the input, the greater the potential for higher forecast accuracy.
Another detail to consider is what forecast model will produce the “best” forecast? Selecting the technology to support demand planning for the organization requires careful consideration. At a minimum, the software should offer multiple forecasting models capable of handling trend, seasonality, and cyclicality. It should be able to handle variable periods of history; self-select the statistically “best” forecast model for the given data set; should identify outliers based on multiple criteria; and allow for top-down and bottom-up forecasting. So, disciplined data collection via controlled processes, input to forecasting software, and enhanced by analysis of outliers will generally produce reasonable, but naïve, statistical forecasts. This enhanced statistical forecast forms the basis of the demand plan.
The naïve forecast is improved when causal influences are considered when analyzing the historical data. Historical demand is affected by “created” events such as having run a marketing promotion, making a close-out sale of excess inventory, or substituting one product for another. Such events cause non-repeating spikes in the historical demand. A change in a customer’s inventory policies allowing them to reduce their inventory levels may create a non-repeating dip in the historical demand. These occurrences must be identified, both through data-driven analysis as well as through collaboration with the parts of the organization that have knowledge of these events. The historical data set must be normalized for the non-repeating scenarios and revised forecasts created in order to improve the accuracy of the future forecast. At this point, the forecast is still reactive; it’s based on what has already happened.
Causal influences must also be built into the future forecast. For example, an upcoming time-bound product promotion could cause a temporary increase in demand, while a permanent price reduction may cause a volatile increase in near term demand before stabilizing. A large customer may publish a notice of a production increase or decrease, or provide a detailed forecast. Such occurrences must be quantified and evaluated for possible inclusion an adjustment to the future forecast.
Experimental methods are used to forecast demand for new products. Developing new item forecasts for inclusion in the overall plan should be a collaboration between Product Marketing and Demand Planning. The forecast may be derived from demand patterns of prior new product introductions, demand patterns of existing similar products, input from customers, or a blend of data from various sources.
The application of judgmental factors to modify the forecast may be prudent in certain scenarios, but carries risk. This is the introduction of extrinsic information to proactively adjust the forecast in anticipation of demand changes. For example, if past demand indicates a strong link between new housing starts and demand for residential windows and other fixtures, it may be reasonable to incorporate a factor for the outlook on new housing starts into the forecast for windows. There should be agreement on which factors will be considered for application to the forecast, and guidelines established to define how much the forecast may be changed at one time without seeking additional input.
The Demand Planning function facilitates the collection of data and information and builds the plan, and measures and reports the accuracy of the overall forecast as well as the accuracy of the intelligence layered into the demand plan. Each contributing function, including Demand Planning, is responsible for their contribution and must be held accountable by management and strive to continuously improve the quality and timeliness of the information they provide.
Effective demand planning requires the engagement of many functions within an organization. It can therefore not simply be an event, a task to complete. It must become a process embedded into the very culture of the organization.